Exploring Multimodal AI, Agentic AI, Retrieval-Augmented Generation, and Customized Enterprise Generative Models
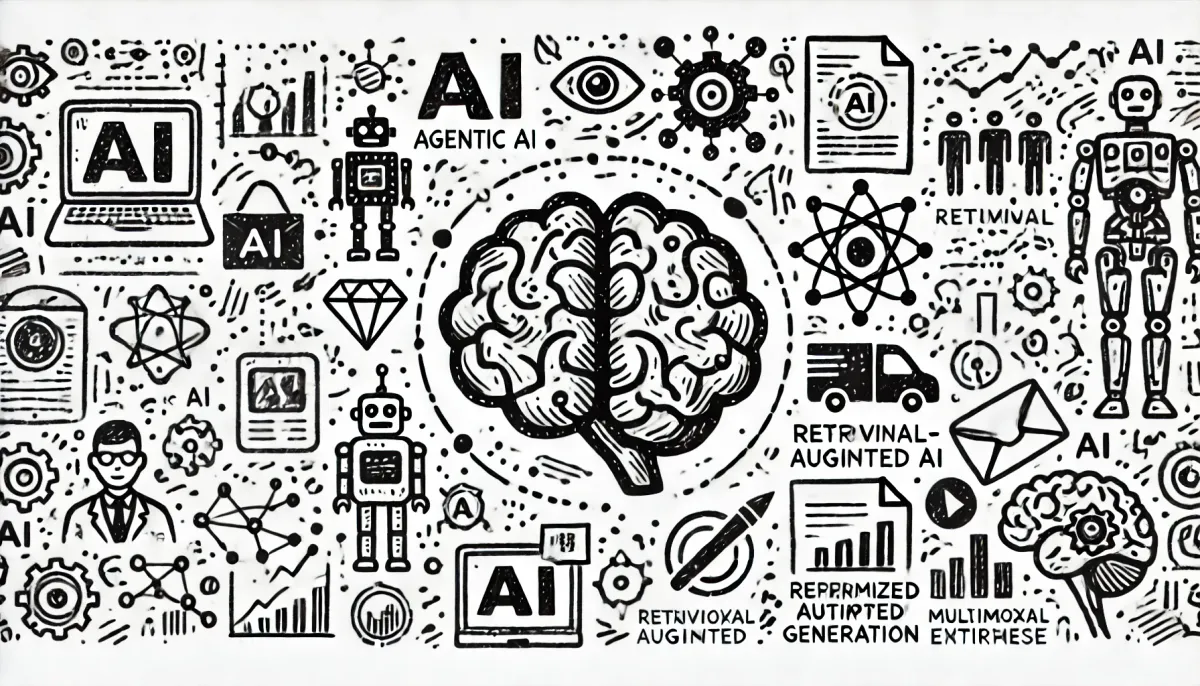
Artificial intelligence is evolving at a breakneck pace, with new technologies emerging that promise to redefine the landscape of both AI capabilities and business applications. Just a few years ago, the focus was on training large language models (LLMs) and grappling with the staggering costs associated with scaling them. Today, the conversation has shifted. The AI world is buzzing with excitement over innovations like multimodal AI, agentic AI, retrieval-augmented generation (RAG), and customized enterprise generative AI models. Let’s take a closer look at these technologies and why they matter.
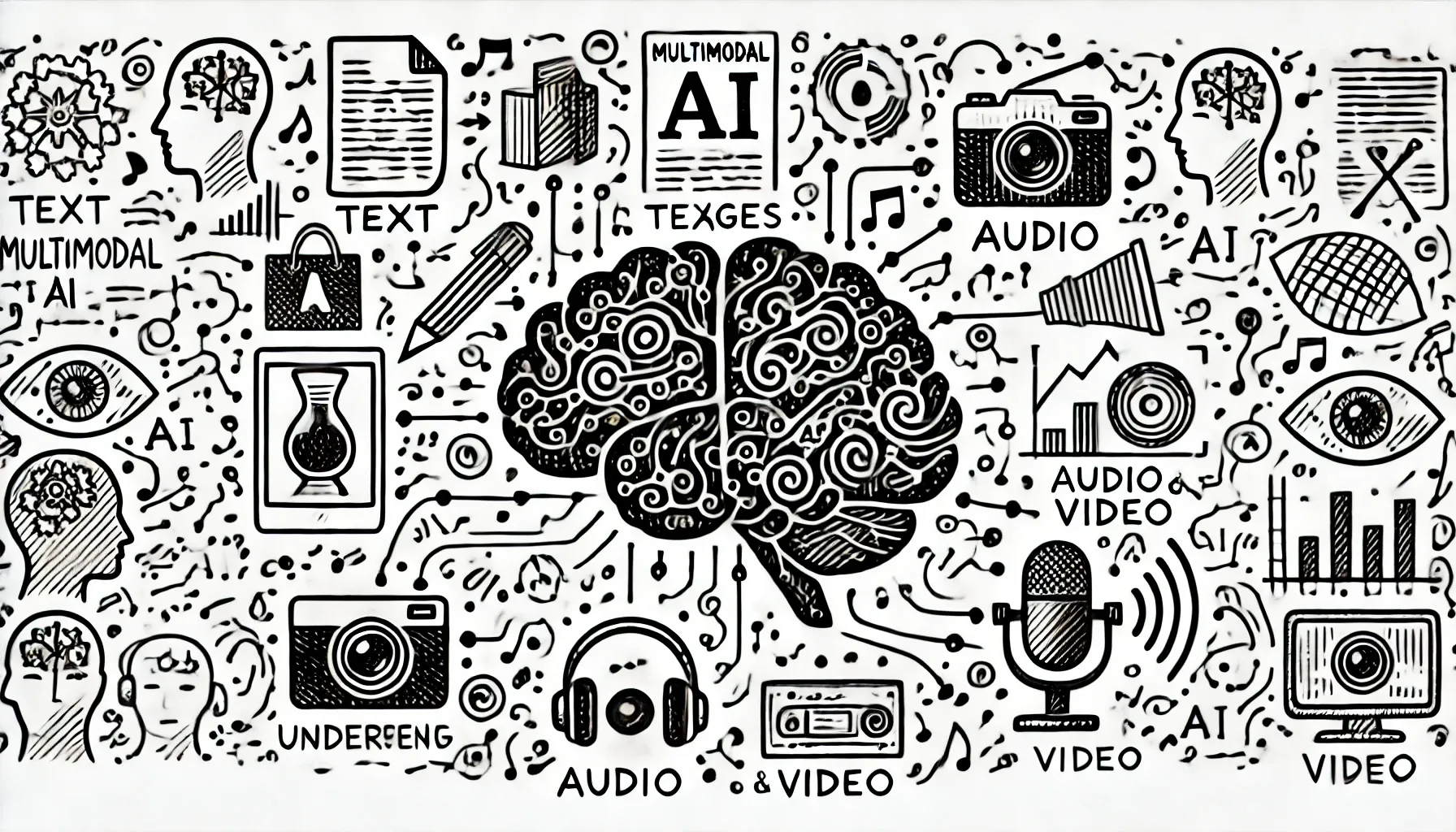
Multimodal AI: Bringing the World Together
We’ve all seen (and marveled at) the power of language models like GPT-4, but the real game-changer is its multimodal capability. Multimodal AI refers to models that can process and understand different types of data—text, images, audio, and video—all in one go. In practical terms, this means that instead of being limited to text-based conversations, AI can now interpret a photo or a diagram alongside text, providing a more nuanced and human-like understanding of information.
Imagine an AI doctor that can read your medical history (text), analyze your MRI scan (image), and detect irregularities in your heartbeat (audio) all at once. Or consider a digital assistant that not only helps you organize your calendar but can also interpret your sketches, identify items in photos, and even understand your mood based on your tone of voice. Multimodal AI allows for a richer, more immersive interaction with technology.
This broadens AI’s utility across various sectors. Multimodal AI isn't just about adding bells and whistles; it allows AI systems to interact with the world more like humans do—using a variety of sensory inputs. In the long run, we’re looking at more powerful tools for healthcare, autonomous systems, and human-computer interaction.
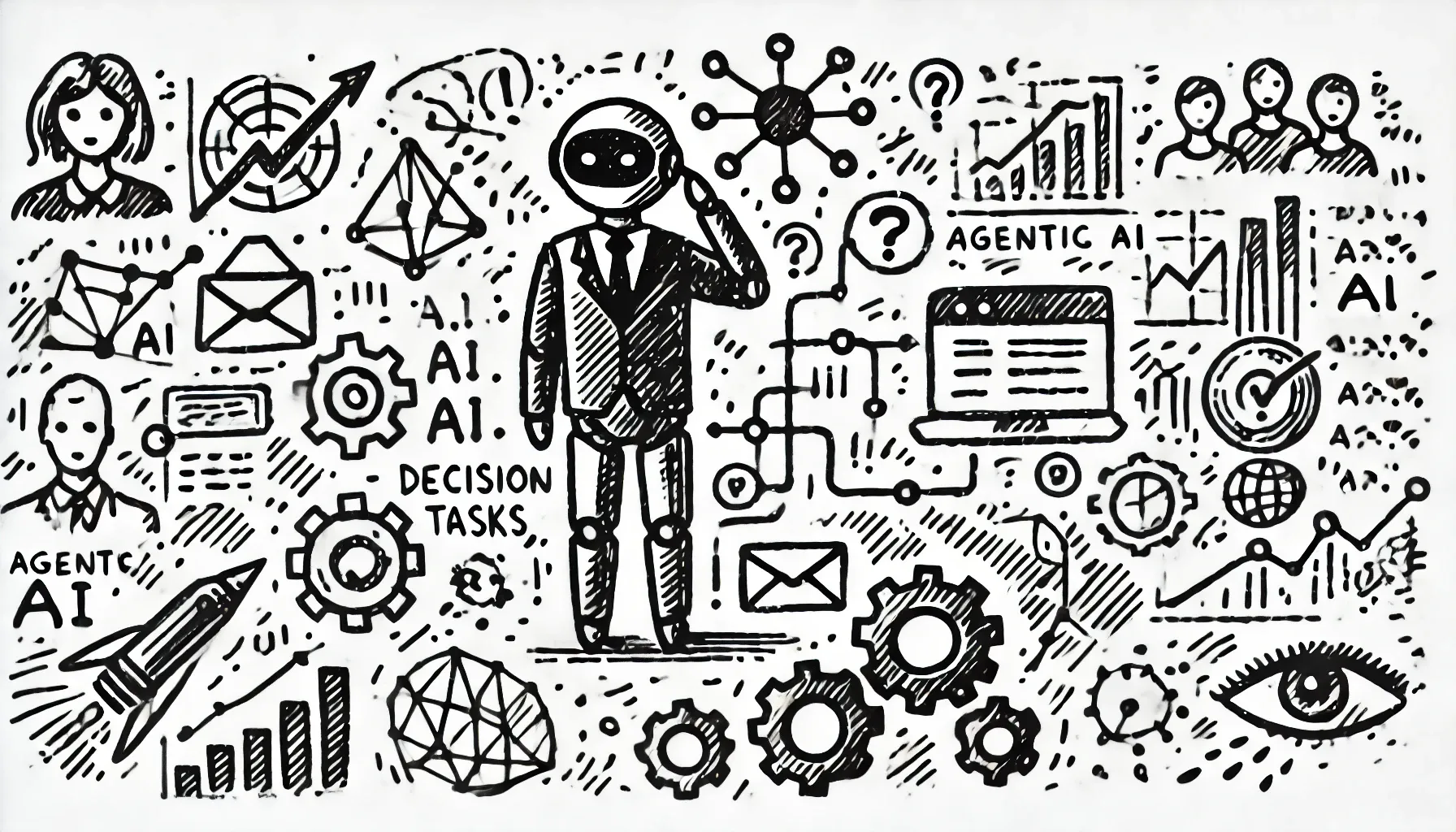
Agentic AI: Giving AI Independence
While AI has made leaps in specific, narrow tasks, it often needs close supervision to stay on track. Enter agentic AI—AI systems designed to act autonomously. This concept takes AI from being a passive tool to one that can actively solve problems and make decisions without constant human input. Think of it as a worker with enough initiative to find solutions on their own, rather than asking for permission every step of the way.
Agentic AI systems can be given a goal—like optimizing a supply chain—and then allowed to explore the most efficient way to achieve it. Along the way, they might reconfigure processes, test out new methods, and refine their strategies, all with minimal human oversight. Essentially, these AI agents exhibit a form of "decision-making agency."
For industries that manage complex, large-scale operations, agentic AI holds the promise of significant cost reductions and efficiency gains. Businesses in logistics, financial trading, or IT infrastructure management could leverage these autonomous systems to handle intricate, time-consuming tasks without the need for a human babysitter.
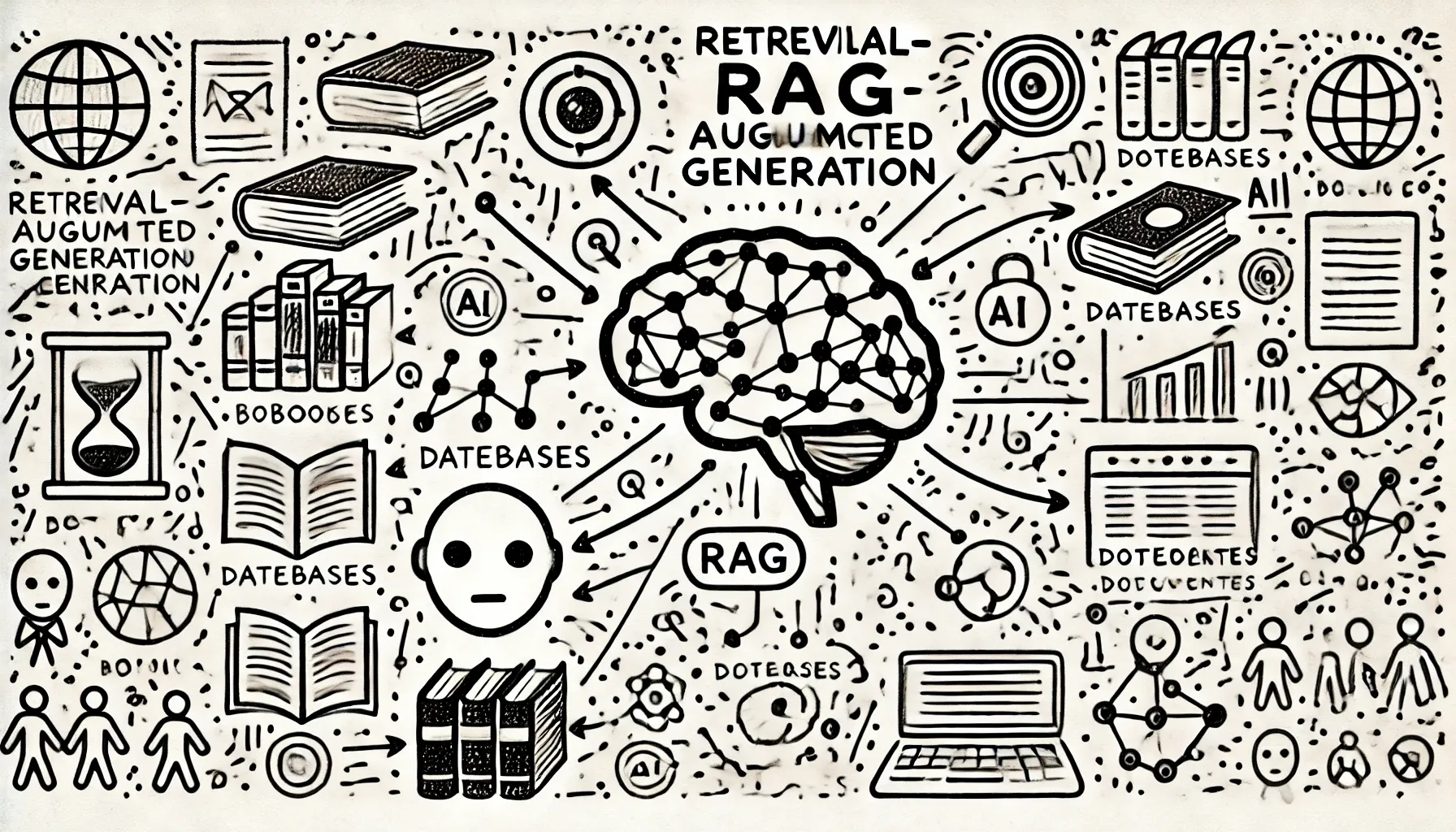
Retrieval-Augmented Generation: Smarter, More Accurate AI
While generative models like GPT-3 and GPT-4 are impressively creative, they often hit a wall when it comes to factual accuracy. This is where Retrieval-Augmented Generation (RAG) comes in. Instead of relying solely on the knowledge packed into its model during training, a RAG system can access external databases, documents, and other resources to provide more accurate, up-to-date responses.
Consider a customer support chatbot powered by RAG. Rather than just drawing on pre-programmed responses or outdated knowledge, it can pull the latest troubleshooting steps from an internal database, ensuring that the information it provides is always current and relevant. The same goes for industries like law and healthcare, where having the most recent and accurate data is crucial.
RAG bridges the gap between AI's generative capabilities and the need for real-time, factual accuracy. This is particularly important in domains like finance, legal, or scientific research, where outdated or incorrect information could lead to serious consequences. By coupling creativity with real-time retrieval, RAG enables AI systems to be both imaginative and reliable.
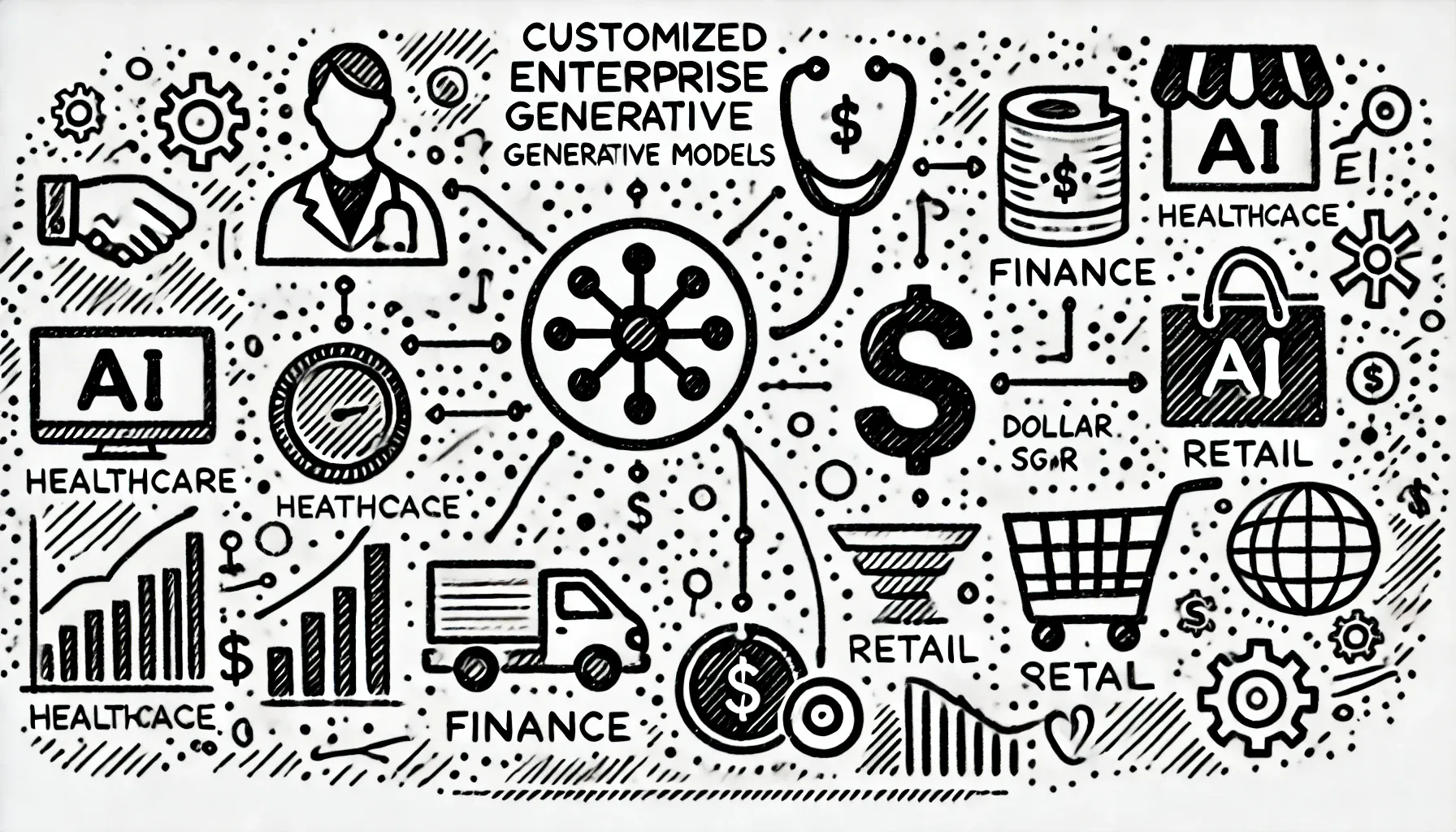
Customized Enterprise Generative AI: Tailoring AI for Business Needs
In recent years, many businesses have realized that while general-purpose AI models are powerful, they often fall short when it comes to meeting specific, niche needs. Enter customized enterprise generative AI models—AI systems designed and trained to work specifically with a company’s proprietary data and workflows.
These models go beyond the “one-size-fits-all” approach of general LLMs. For example, a healthcare provider could develop an AI system trained exclusively on their internal medical records and clinical data, making it far better suited for diagnostics or treatment recommendations than a more generic model. A retail company, on the other hand, might use a custom model trained on their customer purchase patterns, allowing it to generate highly personalized marketing content or optimize inventory management with remarkable accuracy.
Tailored AI models allow businesses to fully unlock the potential of their own data. In highly regulated or competitive industries, where data privacy and specificity are paramount, having a model trained on internal information offers a major advantage. These customized models provide better performance and deeper insights, enabling companies to maintain a competitive edge while ensuring their AI tools are highly relevant to their industry needs.
The Bigger Picture
All four of these technologies—multimodal AI, agentic AI, retrieval-augmented generation, and customized enterprise models—represent significant steps forward in the AI world. They not only demonstrate how far AI has come but also point toward a future where AI systems will be more autonomous, adaptable, and responsive to real-world needs. Whether you're a business leader looking to integrate cutting-edge AI into your operations or simply someone fascinated by the rapid advancement of technology, these innovations signal the next wave of AI’s evolution. And it’s a future that’s coming fast.
Recommended Reading on AI
"Artificial Intelligence: A Guide for Thinking Humans" by Melanie Mitchell - This book provides an accessible yet comprehensive introduction to AI, covering its history, current developments, and future potential. It also explores the limitations and ethical considerations of AI technologies, making it a must-read for anyone interested in understanding AI's broader impact.
"Human Compatible: Artificial Intelligence and the Problem of Control" by Stuart Russell - Russell, a leading AI researcher, discusses the challenges of creating AI systems that are beneficial to humans. The book addresses key concerns around AI safety and the development of agentic AI, making it particularly relevant in the context of autonomous systems.
"Prediction Machines: The Simple Economics of Artificial Intelligence" by Ajay Agrawal, Joshua Gans, and Avi Goldfarb - Focused on the business implications of AI, this book explains how AI technologies, like retrieval-augmented generation and customized enterprise models, are transforming industries. It’s ideal for readers who want to understand how AI impacts decision-making and business processes.
"Rebooting AI: Building Artificial Intelligence We Can Trust" by Gary Marcus and Ernest Davis - A critical look at the current state of AI, focusing on the gaps between expectations and reality. The book discusses the limitations of current AI technologies like multimodal AI and the need for more reliable systems in the future.
"Architects of Intelligence: The Truth About AI from the People Building It" by Martin Ford - In this book, Martin Ford interviews 23 of the most prominent figures in AI research. The discussions provide insight into the cutting-edge developments in AI, including multimodal AI, agentic AI, and enterprise applications.
References:
- OpenAI (2023). "Introducing GPT-4, a Large Multimodal Model." Retrieved from https://openai.com/research/gpt-4This paper explains the development of GPT-4, which introduced multimodal capabilities, processing both text and images.
- Microsoft (2024). "The Future of Autonomous Agents in AI." Retrieved from https://microsoft.com/research/autonomous-aiA detailed discussion on the rise of agentic AI and its applications in various industries.
- Hugging Face (2023). "Exploring Retrieval-Augmented Generation (RAG)." Retrieved from https://huggingface.co/blog/ragThis article dives into the concept of Retrieval-Augmented Generation and how it enhances the factual reliability of AI models.
- McKinsey & Company (2024). "Customizing Generative AI for Enterprise Applications." Retrieved from https://mckinsey.com/reports/generative-aiA comprehensive report on the benefits of building customized generative AI models for enterprise use cases.
- AI Weekly (2024). "Trends in AI: Multimodal and Retrieval-Augmented Systems." Retrieved from https://aiweekly.com/articles/latest-trendsA broad overview of the latest trends in AI, including multimodal systems and customized generative models.